Blog
How manufacturers can use data to improve predictive maintenance
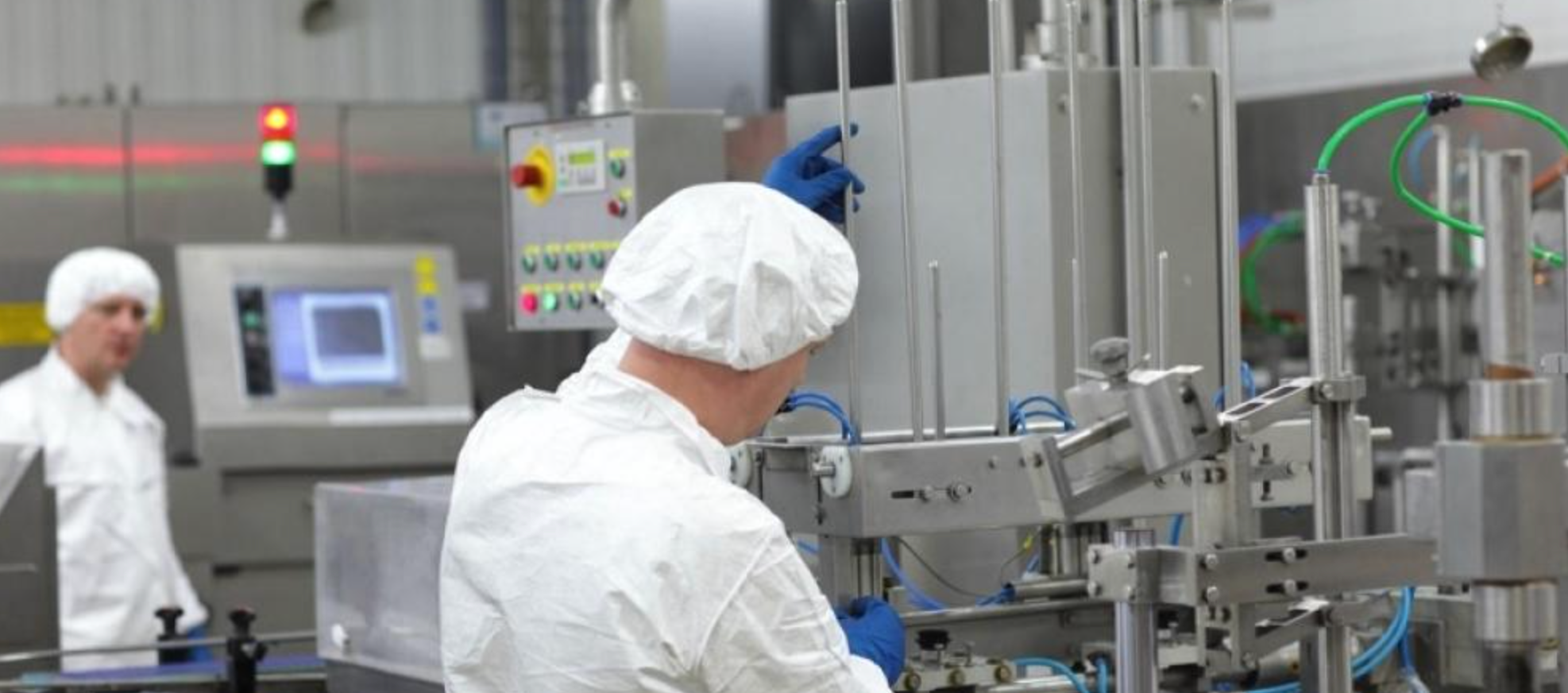
When manufacturing production stops for an unplanned event, such as equipment failure, it accumulates down time. Maintaining equipment and machinery while keeping costs down is a constant challenge for manufacturers. Predictive maintenance uses IoT-based resources, including autonomous mobile robots, to communicate information about the health of equipment and keep production on-line.
The inefficiency of down time
An inefficient maintenance schedule can affect a facility’s overall performance by 5-20%, with studies showing that unscheduled down time costs industrial manufacturers approximately $50 billion per year. Running machinery until failure does maximize utilization but can lead to damage as parts overheat and break. If the part is not on hand when it fails, down time can be pushed days or weeks, leading to a buildup of inventory. Since unscheduled down time can be costly, more frequent service and parts replacement might be a better alternative for some equipment.
The smart factory enables manufacturers to strike a balance between maximizing the useful life of their equipment while minimizing planned down time. Equipment sensors, computerized maintenance management systems, and enterprise resource planning all provide data to make decisions about downtime easier for manufacturers to make. Automated material handling equipment like autonomous mobile robots can contribute data as well with information about performance and as well as their own status. Predictive algorithms lead to better performance as compared to preventative maintenance programs, which use manual data.
How predictive maintenance works
Predictive maintenance technology, which can be installed with existing systems, does not only one type of data, instead, data from multiple sources is used by computer systems.
For example, vibration analysis is commonly used technologies in which internal component faults are identified and quantified. When the failure warning is generated based on the vibrations, repair can be scheduled for non-working hours. Cloud-based algorithms can evaluate millions of similar parts currently in use and predict their life cycle.
The conclusions drawn from the data may instruct the machines to change their functions, or a technician might be alerted to an issue. Predictive algorithms can create a work order in the system, check the ERP system for parts on hand and create a purchase order for any parts that are needed. It can also show which parts wear faster and suggest realignment to optimize machine use.
The benefits of predictive maintenance
Predictive maintenance allows manufacturing facilities to optimize maintenance to maximize the life of the machinery while minimizing the disruption to operations. The benefits of predictive maintenance in a manufacturing setting are plentiful:
Reduces equipment and labor costs: Because a repair is made to a part prior to failure, cost is minimized on both the part and the labor necessary for the repair.
Increased Equipment Uptime: Uptime may increase as much as 10-20%, as parts replacement is scheduled only during downtime, or non-productive times.
Reduced maintenance planning time: Because much of the process is automated, planning time can be cut by 20-50%.
Increased Revenue: With a material cost savings of 5-10%, reduced inventory carrying costs, and repairs that are handled faster with reduced downtime, revenue will increase.
With more time spent on problem resolution driven by data, clear paths for initiatives that can improve performance emerge. Predictive maintenance allows manufacturers to deal with potential problems before they disrupt the production schedule, leading to better overall operations management and productivity within the plant.