Blog
5 AI technologies and their application in material handling

Artificial Intelligence (AI) has and continues to have a seismic impact on logistics, with estimates that the technology could add between $1.3 trillion and $2 trillion a year in value to the industry.
This increase in value will mainly be achieved by driving cost, time and resource efficiencies through ‘smart’ automation. AI is best understood as a collection of related technologies which share the characteristics of combining insight with autonomous action.
Below are five examples of AI technologies and how they are being deployed in the material handling sector today.
1. Autonomous vehicles & robots
Autonomous vehicles and picking robots are probably the most well-known examples of AI used in material handling. The evolving automation of equipment such as forklifts, pallet jacks, and wheeled totes is founded on the use of data analytics, camera, and sensor technologies to take care of decision-making tasks such as navigation path planning and collision avoidance. Similarly, we are now seeing robotic picking arms capable of precision unit sorting tasks which can pick, sort and pack goods based on specifications like weight, shape and branding design.
The level of autonomy such systems have is progressing all the time as the technology develops. For example, while fixed-path autonomous guided vehicles (AGVs) have proved popular across the global material handling sector over the past few years, the evolution of autonomous mobile robots (AMRs) is further boosting the efficiency of material handling, because they can move through a warehouse infrastructure-free and adapt to path changes in real-time.
This increased efficiency is reflected in the growing adoption of automation technologies, with nearly 80% of companies planning to adopt the technology in the next five years, according to the MHI 2023 Annual Industry Report. The report also states that Robotics and Automation is “table stakes for operations to remain competitive,” with 78% of companies stating that the technology has the potential to create competitive advantage or disrupt the industry.
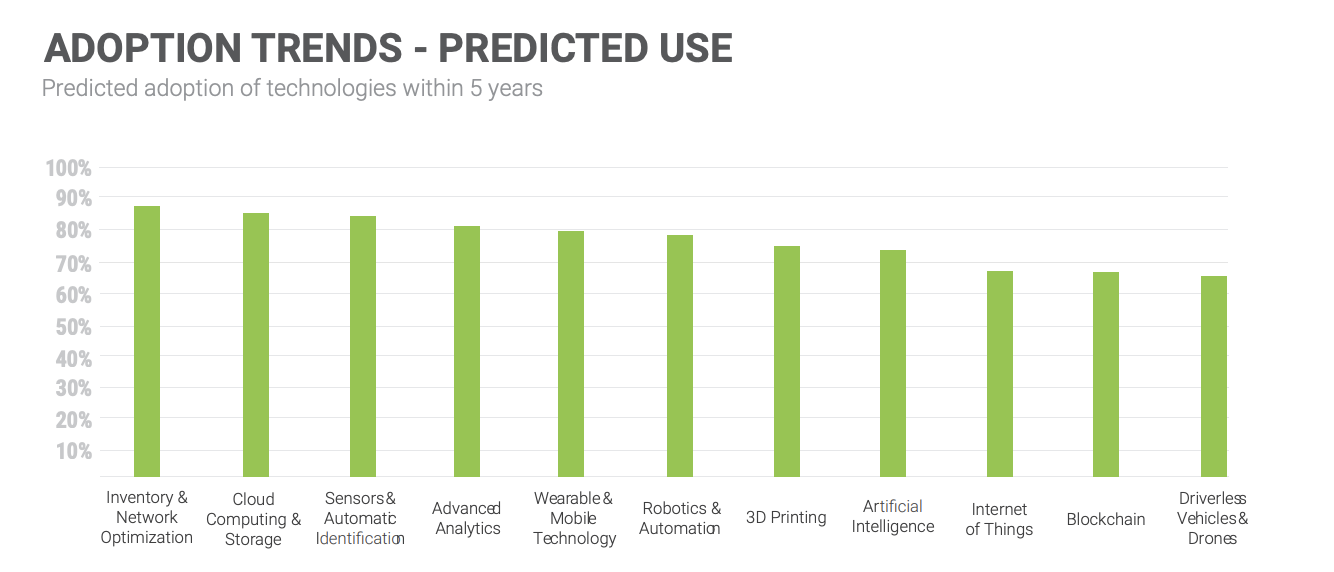
Graph 1: Predicted adoption of key supply chain technologies.
Robot-use continues to grow, which is helping make U.S. companies more competitive and leading to new job growth. We are currently experiencing the greatest period of robot expansion in history—over 180,000 robots have been shipped to American companies since 2010, and more than 1.2 million new manufacturing jobs have been created during this time.
Jeff Burnstein
President, Association for Advancing Automation
2. Computer vision
Computer vision is the branch of AI which involves the interpretation of visual data captured by a camera. For example, computer vision is used by intelligent robot pickers to identify and sort different items based on visual cues, and autonomous robots use computer vision to monitor their surroundings to avoid collisions.
Other applications include visual inspection of goods to automate aspects of quality control. Combined with autonomous vehicles and robotic picking equipment, this technology can go as far as removing damaged or unsatisfactory items from shipments, and has the potential to further extend into inventory management, with AI-powered visual assessment of shelves logging on-shelf availability and stock levels.
3. Natural Language Processing
Natural Language Processing (NLP) is a technology that forms part of what IBM calls “cognitive automation”: the intersection of AI with robotic process automation (RPA) software to provide intelligent, autonomous management of back-office administration tasks.
NLP helps to automate tasks involving documentation and paperwork, allowing the “unstructured” data in text documents to be interpreted and used by a computerized system. This means that documents like invoices and order forms can be processed and checked for anomalies in seconds, which is important for businesses that are handling shipments to and from multiple different sources and have to cross-reference large quantities of paperwork.
4. Conversational interfaces
Another side to NLP technology is its ability to process and interpret the spoken word, which in turn opens the door to what are known as conversational interfaces. Just as our homes are increasingly being filled with devices we can activate using voice commands, conversational interfaces are being added to warehouse management systems to provide a point of contact between human workers and machines. Human-to-machine information exchanges can become much richer and more intuitive, with commands not restricted to a narrow list of keywords, and automated systems that are able to provide detailed spoken answers to questions rather than just churn out data tables.
While executives are excited about the efficiency possibilities of conversational interfaces, workers are also accepting of the innovation, with 60% saying they could easily imagine being supported by a conversational robotic colleague.
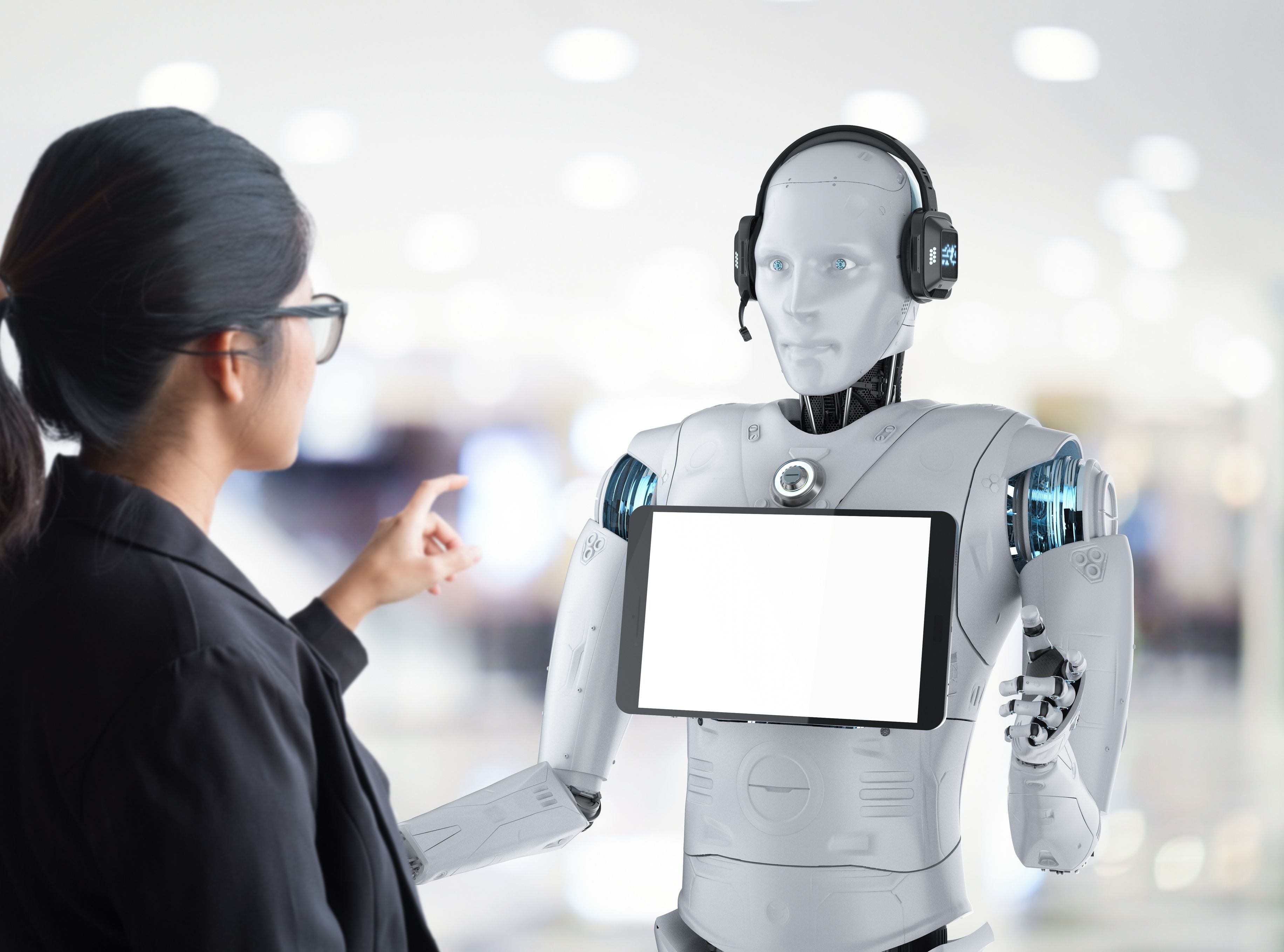
Image 1: Collaboration between workers and conversational robotic technology is the future of AI, and is already being developed today.
5. Machine Learning
Machine Learning is a technology which underpins all of the AI use cases above. Through the use of algorithms, capable of modifying and adapting their own outputs over time based on interpreting patterns in data, Machine Learning is the branch of AI that allows automation to become truly autonomous by optimizing and adjusting its own performance.
Therefore, it is Machine Learning that allows an AMR in a warehouse to constantly recalibrate the optimum navigation path and picking order to keep driving incremental efficiency gains. Machine Learning also enables picking robots, visual inspection machines and document processing RPAs to not only spot anomalies quickly, but also determine the reasons behind them independently by interrogating end-to-end system data.